Snowfall Prediction Models: Understanding The Science Behind Forecasting Winter Weather
Snowfall prediction models are critical tools for meteorologists in forecasting winter weather patterns. These models help predict the amount, timing, and location of snowfall, ensuring communities are prepared for potential disruptions. As climate change continues to impact weather patterns, the accuracy and reliability of these models have become increasingly important.
Winter weather can have significant consequences, from transportation disruptions to power outages and even public safety risks. Accurate snowfall predictions play a pivotal role in mitigating these impacts by allowing governments, businesses, and individuals to take proactive measures. This article delves into the intricacies of snowfall prediction models, their applications, and the advancements shaping this field.
In the following sections, we will explore the science behind snowfall prediction, the different types of models used, and how these models contribute to effective decision-making. Whether you're a meteorology enthusiast or a stakeholder in weather-related planning, this article provides a comprehensive overview of the tools and technologies driving winter weather forecasting.
Read also:Unveiling The Mystique Of Alina Angel A Rising Star In The Spotlight
Table of Contents
- Introduction to Snowfall Prediction Models
- Types of Snowfall Prediction Models
- Key Components of Snowfall Prediction Models
- Applications of Snowfall Prediction
- Limitations and Challenges
- Advancements in Technology
- Case Studies
- Data Sources and Reliability
- Future Trends in Snowfall Prediction
- Conclusion and Next Steps
Introduction to Snowfall Prediction Models
Snowfall prediction models are sophisticated systems designed to forecast snow accumulation and distribution. These models rely on complex algorithms, meteorological data, and atmospheric physics to estimate snowfall patterns. Understanding the fundamentals of these models is essential for anyone interested in meteorology or climate science.
How Snowfall Models Work
Snowfall prediction models operate by analyzing various atmospheric variables, such as temperature, humidity, wind speed, and pressure. These variables are processed through numerical weather prediction (NWP) systems, which simulate atmospheric conditions over time. The models then generate outputs that predict the likelihood, timing, and intensity of snowfall events.
Why Snowfall Prediction is Important
Accurate snowfall predictions are crucial for public safety, infrastructure management, and economic planning. For instance, municipalities rely on these forecasts to prepare for snow removal operations, while airlines use them to schedule flights during winter months. The ability to predict snowfall with precision can save lives and reduce financial losses.
Types of Snowfall Prediction Models
There are several types of snowfall prediction models, each designed for specific purposes and conditions. These models vary in complexity, scope, and application, offering a range of options for meteorologists and researchers.
Global vs. Regional Models
- Global Models: These models cover large geographical areas and provide broad forecasts. Examples include the Global Forecast System (GFS) and the European Centre for Medium-Range Weather Forecasts (ECMWF).
- Regional Models: Focused on specific regions, these models offer higher resolution and more detailed predictions. Examples include the North American Mesoscale Model (NAM) and the Rapid Refresh Model (RAP).
Statistical vs. Dynamical Models
- Statistical Models: Use historical data and statistical techniques to predict snowfall patterns. These models are often simpler and faster but may lack the precision of dynamical models.
- Dynamical Models: Simulate atmospheric processes using physical equations. These models are more complex and computationally intensive but provide more accurate forecasts.
Key Components of Snowfall Prediction Models
To function effectively, snowfall prediction models incorporate several key components. These components work together to ensure the accuracy and reliability of the forecasts.
Atmospheric Data
Models rely on a wide range of atmospheric data, including temperature, humidity, wind speed, and pressure. This data is collected from various sources, such as weather stations, satellites, and aircraft.
Read also:Girthmaster And Miaz A Deep Dive Into The Phenomenon You Need To Know
Initial and Boundary Conditions
Initial conditions refer to the current state of the atmosphere, while boundary conditions represent the external influences affecting the model's domain. Both are critical for ensuring the model's accuracy and stability.
Applications of Snowfall Prediction
Snowfall prediction models have numerous practical applications across various sectors. From transportation to agriculture, these models play a vital role in decision-making processes.
Transportation and Infrastructure
Accurate snowfall forecasts help transportation authorities plan for road closures, snow removal operations, and traffic management. Similarly, utility companies use these predictions to prepare for potential power outages and infrastructure damage.
Agriculture and Water Management
Farmers rely on snowfall predictions to plan planting schedules and irrigation strategies. Additionally, water resource managers use these forecasts to estimate snowmelt runoff and manage reservoir levels.
Limitations and Challenges
Despite their sophistication, snowfall prediction models face several limitations and challenges. These challenges can impact the accuracy and reliability of the forecasts, necessitating ongoing research and development.
Data Gaps and Uncertainty
Insufficient data in certain regions, such as remote or mountainous areas, can lead to inaccuracies in model outputs. Additionally, uncertainties in atmospheric conditions and model parameters can affect forecast reliability.
Complexity of Atmospheric Processes
The atmosphere is a highly complex system with numerous interacting variables. Capturing all these variables in a model is challenging, leading to potential errors in predictions.
Advancements in Technology
Recent advancements in technology have significantly improved the capabilities of snowfall prediction models. From improved data collection methods to enhanced computational power, these innovations are driving progress in the field.
Machine Learning and Artificial Intelligence
Machine learning algorithms and artificial intelligence are increasingly being integrated into snowfall prediction models. These technologies enable models to learn from historical data and improve their accuracy over time.
High-Resolution Modeling
Advances in computational power have made high-resolution modeling more feasible. These models provide more detailed and precise forecasts, particularly in complex terrain such as mountainous regions.
Case Studies
Several case studies highlight the effectiveness and limitations of snowfall prediction models in real-world scenarios. These studies provide valuable insights into the practical applications of these models.
Blizzard of 2016
The Blizzard of 2016 was one of the most significant winter storms in U.S. history. Snowfall prediction models played a crucial role in forecasting the storm's path and intensity, enabling authorities to take appropriate measures.
European Winter Storms
In Europe, snowfall prediction models have been instrumental in managing winter storms that affect transportation and infrastructure. Case studies from recent years demonstrate the models' effectiveness in mitigating storm impacts.
Data Sources and Reliability
The reliability of snowfall prediction models depends heavily on the quality and availability of data. Understanding the sources of this data and their limitations is essential for interpreting model outputs.
Satellite Observations
Satellites provide valuable data on atmospheric conditions, including temperature, humidity, and cloud cover. However, satellite data can be limited by factors such as cloud cover and instrument accuracy.
Ground-Based Measurements
Ground-based weather stations offer direct measurements of atmospheric variables, providing critical data for model initialization. However, the distribution and density of these stations can vary significantly across regions.
Future Trends in Snowfall Prediction
The future of snowfall prediction models looks promising, with ongoing research and technological advancements driving innovation. These trends are expected to enhance the accuracy and reliability of forecasts.
Integration of Multi-Model Systems
Multi-model systems combine outputs from multiple models to provide more robust and reliable forecasts. This approach leverages the strengths of individual models while mitigating their weaknesses.
Climate Change Impacts
As climate change continues to alter weather patterns, snowfall prediction models will need to adapt to these changes. Research into the impacts of climate change on snowfall patterns will be critical for improving model accuracy.
Conclusion and Next Steps
Snowfall prediction models are indispensable tools for forecasting winter weather and mitigating its impacts. By understanding the science behind these models and their applications, we can better prepare for the challenges posed by winter storms.
We encourage readers to explore further resources on snowfall prediction and stay informed about advancements in the field. Additionally, feel free to share your thoughts and questions in the comments section below. For more in-depth information, consider exploring related articles on our website.
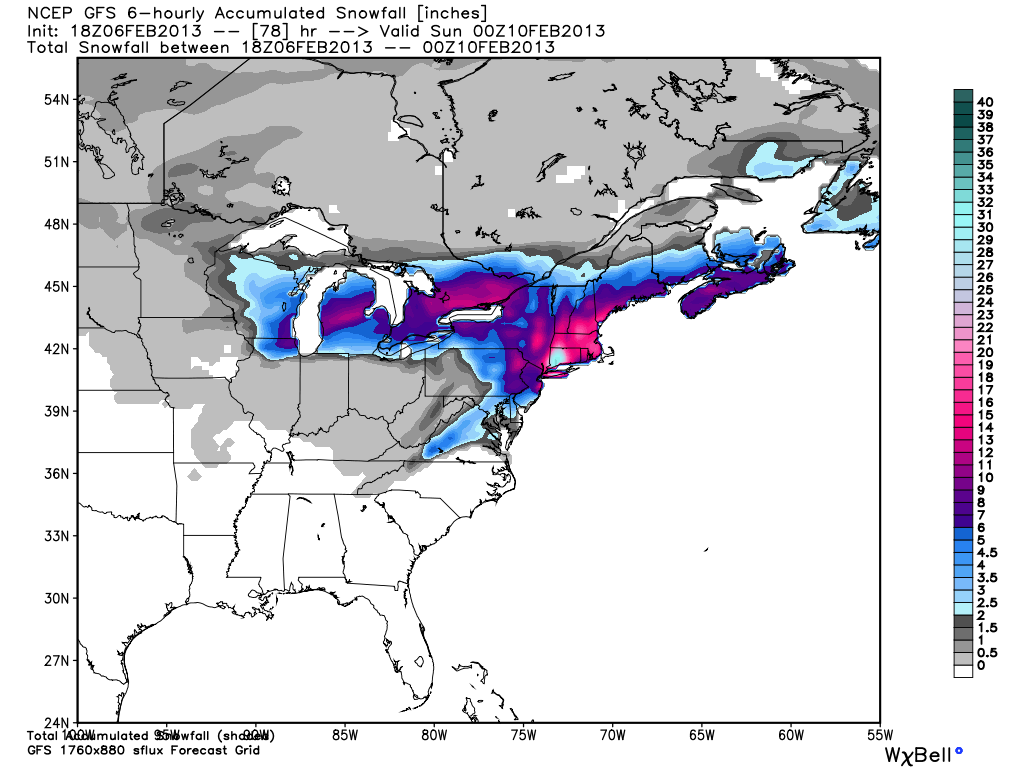

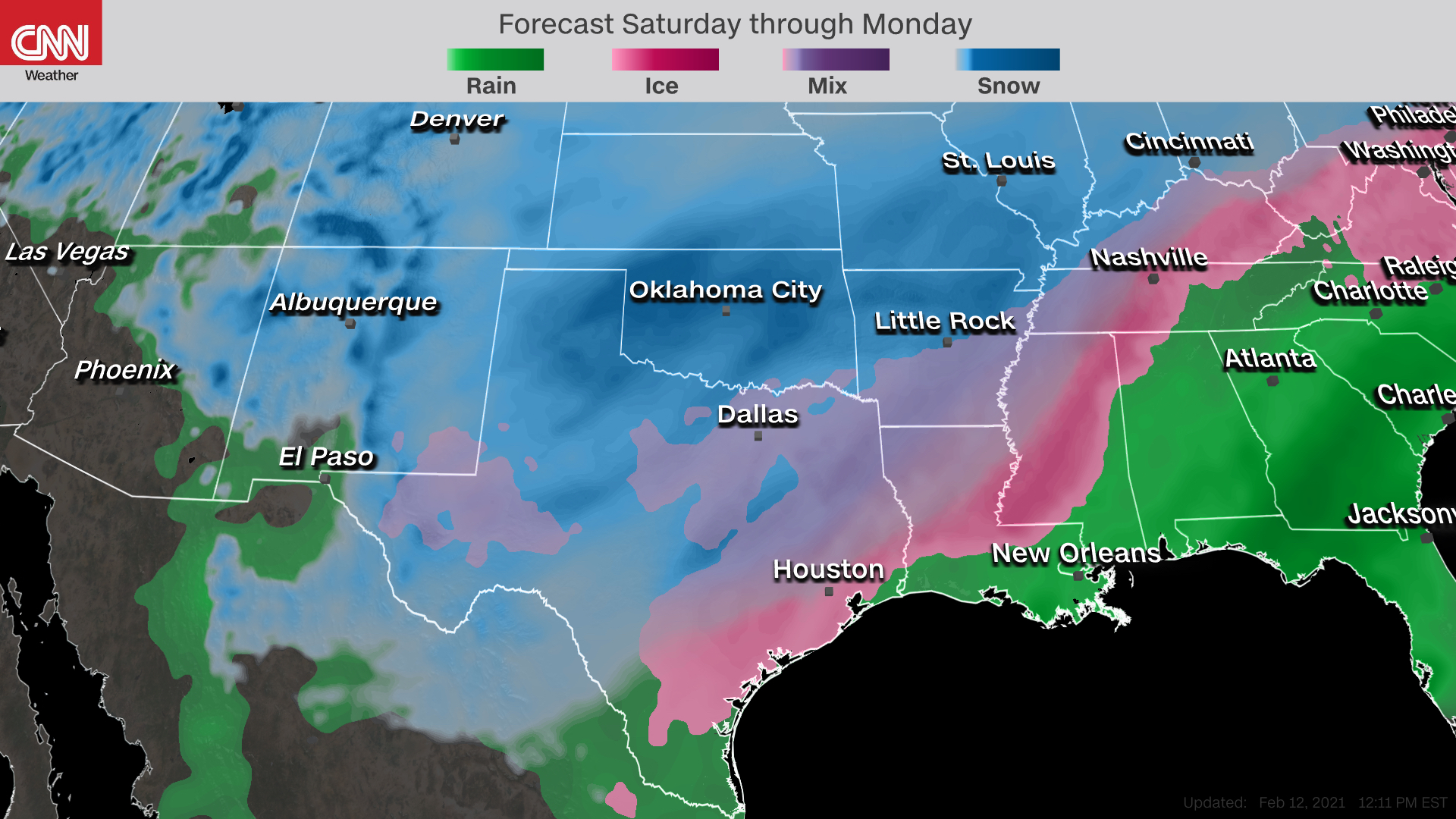